The Role of Machine Learning Data Labeling in Today's Business Landscape
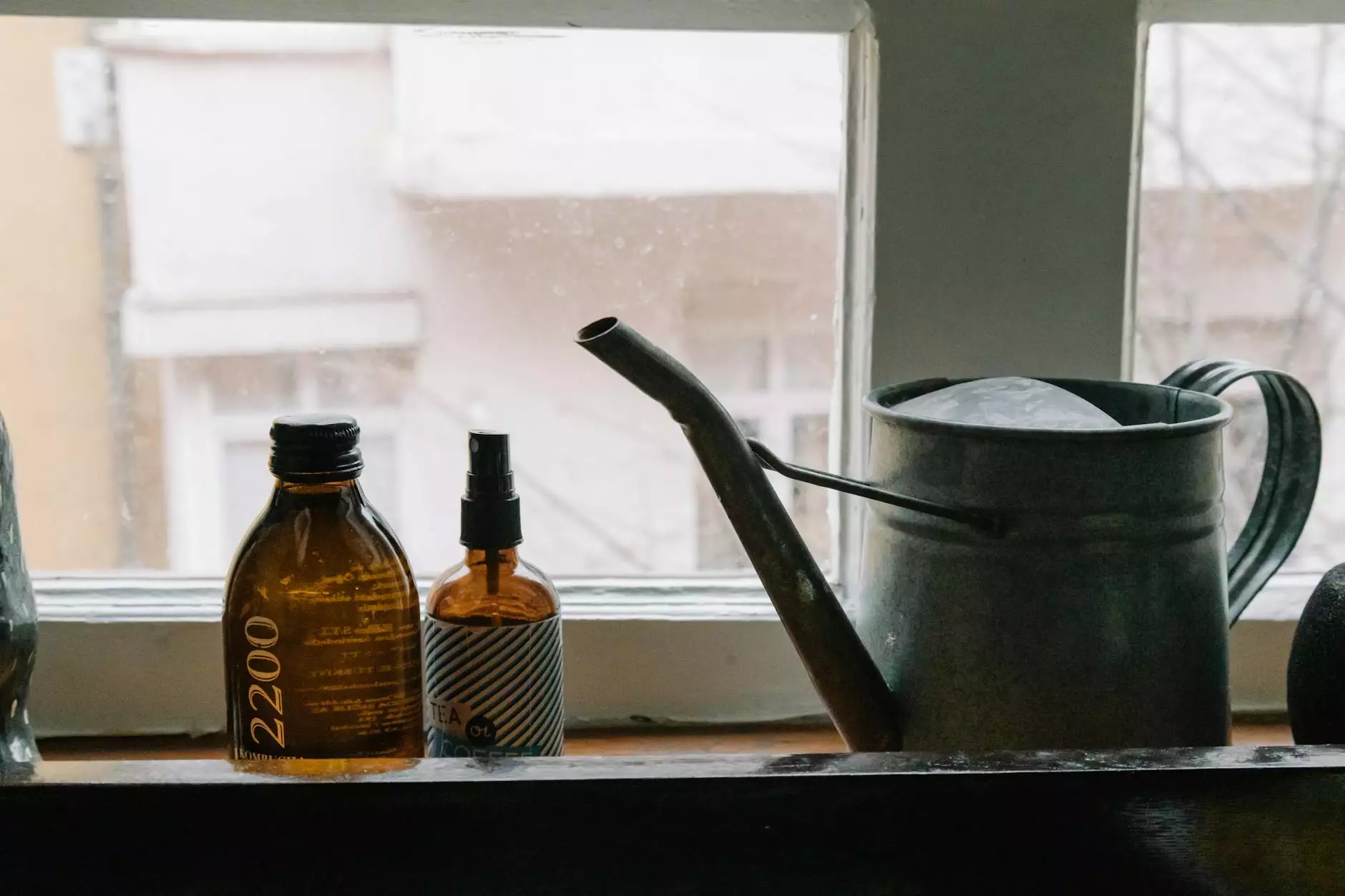
In a rapidly evolving digital world, businesses are leveraging advanced technologies like machine learning to streamline operations, enhance customer experiences, and improve decision-making processes. One of the critical components that underpins successful machine learning initiatives is data labeling. This article explores the significance of machine learning data labeling, particularly within sectors like home services, keys, and locksmiths, and how organizations can benefit from it.
Understanding Machine Learning Data Labeling
Machine learning data labeling refers to the process of annotating data to provide context that machine learning models can use to learn and make decisions. In simple terms, it is the method of categorizing or tagging data points with relevant information. The labeled data then serves as the foundation for training machine learning algorithms, allowing them to recognize patterns and carry out predictive analyses.
Importance of Data Labeling in Machine Learning
The accuracy and effectiveness of machine learning models heavily rely on the quality of the labeled data. Here are several reasons why data labeling is vital:
- Improves Model Accuracy: Well-labeled data enhances the model's ability to make accurate predictions.
- Facilitates Better Training: Labeled datasets provide the necessary feedback for algorithms, ensuring they learn the right outcomes.
- Enables Supervised Learning: Many machine learning techniques require labeled data to train effectively, making labeling essential.
- Streamlines Operation: Labeling data can lead to automatization, aiding in quick decision-making and operational efficiency.
Applications of Machine Learning Data Labeling in Business
Within various business sectors, notably in home services and locksmiths, machine learning data labeling plays a transformative role:
1. Home Services
Home service providers, such as plumbers, electricians, and cleaning services, can benefit from machine learning models powered by accurately labeled data:
Improving Customer Support
Data labeling enables customer service systems to categorize inquiries and complaints accurately. By training models on labeled data, businesses can provide quick and effective responses to customer needs.
Optimizing Service Delivery
Through analyzing labeled datasets, businesses can identify which services are most in demand in particular regions, allowing them to optimize resource allocation.
2. Keys and Locksmiths
The locksmith industry is increasingly adopting machine learning to enhance security solutions.
Predictive Maintenance
Using machine learning algorithms trained on labeled data, locksmiths can predict when a lock may fail or require maintenance, thus improving customer satisfaction and safety.
Enhancing Security Features
By labeling various data points related to lock security, businesses can develop smarter locks that learn from user behavior and adapt to enhance security measures.
Challenges and Solutions in Machine Learning Data Labeling
Despite its importance, machine learning data labeling comes with its challenges:
1. Quality of Data
Ensuring high-quality labeled data is paramount. Poorly labeled datasets can lead to inaccurate machine learning models.
2. Cost and Time Implications
Data labeling can be resource-intensive. Companies must allocate time and budget to ensure their datasets are appropriately labeled.
Solutions
To mitigate these challenges, businesses can:
- Invest in robust data labeling tools that facilitate the accurate acquisition of labeled data.
- Utilize crowd-sourcing platforms to obtain labeled data from a diverse workforce.
- Develop a strong internal protocol for data labeling to maintain consistency and accuracy.
Future Trends in Machine Learning Data Labeling
As technology continues to evolve, the future of machine learning data labeling looks promising. Here are some emerging trends:
1. Automation of Data Labeling
With advancements in AI, we are witnessing the rise of automated data labeling tools, which can significantly reduce the time and cost associated with manual labeling.
2. Enhanced Collaboration between Humans and Machines
The future will likely see increased collaboration between human annotators and machine learning algorithms, where humans will oversee and correct AI-generated labels.
3. Real-Time Data Labeling
As businesses strive for quick decision-making, real-time data labeling solutions will become essential, allowing organizations to adapt to changes instantaneously.
Concluding Thoughts
Data labeling is a critical step in the machine learning pipeline that cannot be overlooked. For businesses in the home services and locksmith sectors, investing in effective machine learning data labeling processes can unlock significant operational efficiencies and enhance service quality. As we look to the future, embracing the trends and innovations in data labeling will inevitably allow businesses to thrive in an increasingly competitive landscape.
In summary, the importance of machine learning data labeling cannot be understated. It is the lifeblood of successful machine learning applications that drive modern business innovation and improve customer satisfaction. Explore how these insights can shape your business strategies today.